- 重要日期
- 截稿日期: 延期至 2019年4月22日
- 会议日期:2019年5月29-31日
- 录用通知:投稿后20-40天
- 论文出版:收到最终稿后15-20天
- 联系我们
- 邮箱:huiyi123net_may01@126.com
- 手机:0086-18101720867
- 座机:021-51098086
- QQ:2934920393
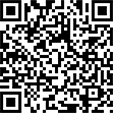
huiyi123公众号
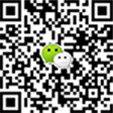
huiyi123微信客服
ICAMDS2019演讲嘉宾信息如下:
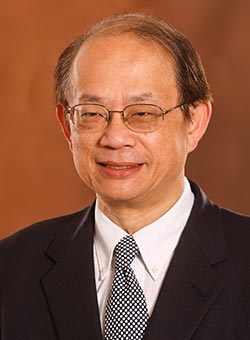
Dr. En-Bing Lin , Professor
Department of Mathematics, Central Michigan University, USA
Biography: Dr. En-Bing Lin is Professor of Mathematics at Central Michigan University, USA. He is a former mathematics department chair at the University of Toledo and Central Michigan University. He has taught and visited at several institutions including Massachusetts Institute of Technology, University of Wisconsin-Milwaukee, University of California, Riverside, University of Toledo, UCLA, and University of Illinois at Chicago. He received his Ph. D. in Mathematics from Johns Hopkins University. His research interests include Data Analysis, Image Processing, Applied and Computational Mathematics, Wavelet Analysis and Applications, and Mathematical Physics. He has supervised a number of graduate and undergraduate students. Dr. Lin serves on the editorial boards of several mathematics and computational journals. He has served on several academic committees of regional and national associations. He has organized several special sessions at regional IEEE conference and American Mathematical Society national and regional meetings. He received Central Michigan University Distinguished Service Award and many research, travel and education grants.
Topic: Incomplete Information Systems and Big Data Analytics
Abstract: We provide an overview of Big Data Analysis and current trends of data analytics to process information systems. Rough Set Theory (RST), which was introduced by Pawlak in 1982 to deal with data analysis based on approximation methods in information systems. RST is a novel approach to cope with imperfect data analysis as well. From the viewpoint of RST, it is usually to represent a data set of an information system as a table. We discuss the decision tables that some attribute values are lost or incomplete. We show how we pass from classical rough set theory to variable precision generalized rough set theory. RST has many applications in many different areas, such as engineering, environment, banking, medicine, bioinformatics, pattern recognition, data mining, machine learning and others. RST is intrinsically a study of equivalence relations on the universe (a set of objects). In fact, rough sets can be used to represent ambiguity, vagueness and general uncertainty. Given some relations between objects in the set, we can construct lower and upper approximations of the objects. We can also use some advanced computing technologies to determine lower and upper approximations and find several properties of the characteristics of objects within RST, as well as to extend RST to generalized RST. We point out the connection with fuzzy sets, fuzzy binary relations and multiple universal sets. We will also mention some examples and applications.
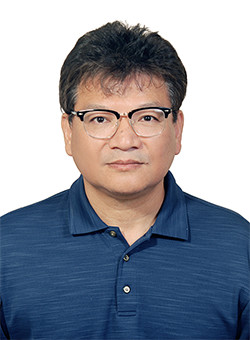
Dr. Feng-Jen Yang, Associate Professor
Computer Science Department, Florida Polytechnic University, USA
Biography: Dr. Feng-Jen Yang received the B.E. degree in Information Engineering from Feng Chia University, Taichung, Taiwan, in 1989, the M.S. degree in Computer Science from California State University, Chico, California, in 1995, and the Ph.D. degree in Computer Science from Illinois Institute of Technology, Chicago, Illinois, in 2001, respectively. Currently, he is teaching and conducting researches at the Florida Polytechnic University. Besides the currently academic career, he also has highly regarded prior research experiences. He once was a research assistant at the Chung Shan Institute of Science and Technology (CSIST), Taoyuan, Taiwan, from 1989 to 1993, as well as an engineer at the Industrial Technology Research Institute (ITRI), Hsinchu, Taiwan, from 1995 to 1996. His research areas include Artificial Intelligence, Machine Learning, Database Management Systems, Data Communications, and Software Engineering. He had also been awarded with research grants from ACM SIGCSE and Seed Grants from Florida Polytechnic University.
Topic: The Implementation of Probabilistic Inferencing and Classification in Python Programming Language
Abstract: Our daily life is full of uncertainty, it is almost inevitable that we have to strive for performing informed behaviors despite the uncertain environment that we are living in. As a result, many intelligent systems are counting on probabilistic inference to support for decisions, hypotheses, forecasts and diagnoses. In this talk, we will go through the mathematic rationale as well as the design and implementation of a probabilistic inference engine. This inference engine is well modeled and implemented by using object-oriented approach. It can be easily plugged into future systems to speed up the development life cycle.